ISSPA 2024 Partner
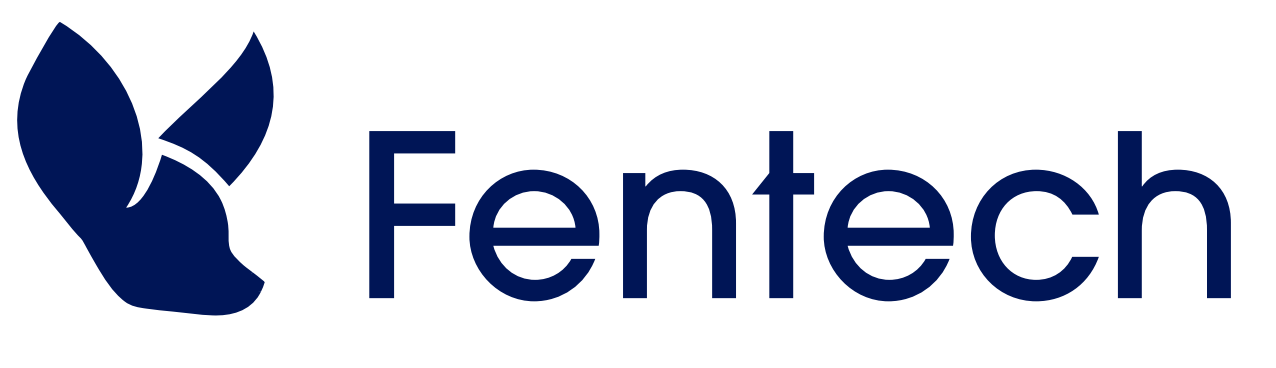
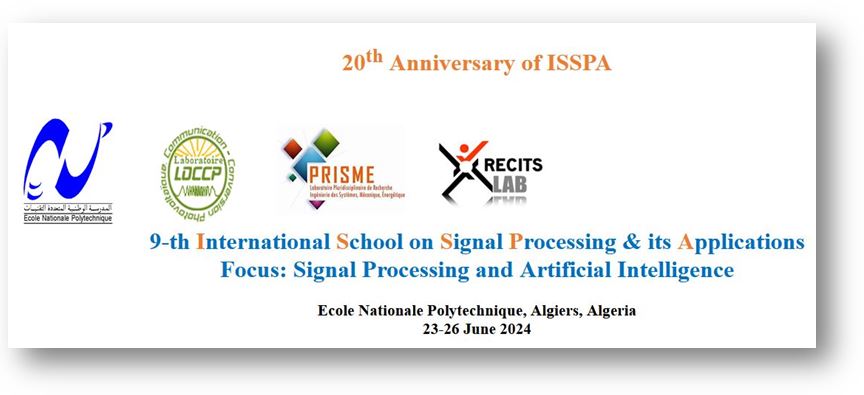
Honorary General Chair
Prof. Abdelouahab MEKHALDI, Ecole Nationale Polyetchnique, Algeria
General Chair
Prof. Adel BELOUCHRANI, Ecole Nationale Polyetchnique, Algeria
Advisory Board
Prof. Adel BELOUCHRANI, Ecole Nationale Polytechnique, Algeria
Prof. Karim ABED-MERAIM, University of Orleans, France
Prof. Nguyen LINH TRUNG, Vietnam National University, Vietnam
Background
The International School on Signal Processing and its Applications has been set up in 2004 to promote signal processing research activities in developing countries, starting with the Maghreb region. This school is dedicated to signal and image processing research. It is a forum that gathers leading researchers and postgraduate students from universities and industrial groups involved in the development of new applications and/or carrying out common research projects.
The first edition of the school took place at Ecole Nationale Polytechnique (ENP), Algiers, in 2004. It has been followed by 5 successive editions organized in Mostaghanem (2005), Jijel (2006), Boumerdes (2007), Kuala Lumpur (Malaysia 2008), and Oran (2009), respectively. After that, the organisation of this thematic school has been resumed in 2017 where it has been organized at the University of Guelma followed by the last edition organized at the University of Annaba in 2019. Several areas related to signal processing are of interest and have been the focus of previous issues.
The 9-th Edition
The focus of this 9-th edition will be on Signal Processing and Artificial Intelligence. Indeed, AI became in the last recent years one of the hottest topics in data science including signal processing.
This new edition celebrates the 20th Anniversary of ISSPA at Ecole Nationale Polytechnique, Algiers, Algeria, and its first place!
Potential Candidates
should send their CV together
with their Poster Title to:
Submission Deadline: 15 April 2024
New Submission Deadline: 30 April 2024
Acceptance Notification: 8 May 2024
Accepted candidates will present
their research work at a POSTER session
Organizing Committee:
Prof. Hacéne BELBACHIR, University of Science and Technology Houari Boumediene, Algeria
Dr. Nesrine BOUADJENEK, Ecole Nationale Polyetchnique, Algeria
Ouiza SATOUR, Ecole Nationale Polyetchnique, Algeria
Souhila KOUICI, Ecole Nationale Polyetchnique, Algeria
Mahieddine DOUADI, Ecole Nationale Polyetchnique, Algeria
Abdelkrim SAHEB, Ecole Nationale Polyetchnique, Algeria
Elies SAADAOUI, Ecole Nationale Polyetchnique, Algeria
Mohamed TAGHI, Ecole Nationale Polyetchnique, Algeria
Sabiha BELOUED, Ecole Nationale Polyetchnique, Algeria
Detailed Program :
Sunday 23 June | Monday 24 June | Tuesday 25 June | Wednesday 26 June |
8:00 am – 8:30 am | |||
Registration | |||
8:30 am – 9:00 am | |||
Opening Ceremony | |||
9:00 am – 10:30 am | 9:00 am – 10:30 am | 9:00 am – 10:30 am | 9:00 am – 10:30 am |
Lecture 1 | Lecture 3 | Lecture 5 | Lecture 7 |
Artificial intelligence and its applications | Divide-and-Conquer Posterior Sampling for Denoising Diffusion Priors | Large Language Models: Challenges and Opportunities | Signal and Information Processing Methods for Brain Studies |
(Prof. Sid-Ahmed Berrani, National School of Artificial Intelligence – Sidi Abdallah) | (Prof. Eric Moulines, Ecole Polytechnique, Paris, France) | (Prof. Mèrouane Debbah, Khalifa University, UAE) | (Prof. Nguyen Linh Trung, VNU, Vietnam) |
10:30 am – 11:00 am | 10:30 am – 11:00 am | 10:30 am – 11:00 am | 10:30 am – 11:00 am |
Coffee Break | Coffee Break | Coffee Break | Coffee Break |
11:00 am – 12:30 pm | 11:00 am – 12:30 pm | 11:00 am – 12:30 pm | 11:00 am – 12:30 pm |
Lecture 2 | Lecture 4 | Lecture 6 | Closing ceremony |
Graph theory and its Applications | Tensor decomposition for neural network | Source Separation: Model driven versus Data driven approaches | |
(Prof. Hacéne Belbachir, USTHB, Algeria) | (Prof. Karim Abed-Meraim, University of Orleans, France) | (Prof. Adel Belouchrani, ENP, Algeria) | |
12:30 pm – 1:30 pm | 12:30 pm – 1:30 pm | 12:30 pm – 1:30 pm | |
Lunch | Lunch | Lunch | |
2:00 pm – 4:00 pm | 2:00 pm – 4:00 pm | ||
Poster Session | Social Activity | Poster Session |
Summaries and speaker’s short biographies
Speaker 1: Prof. Sid-Ahmed BERRANI, National School of Artificial Intelligence, Sidi Abdallah, Algeria
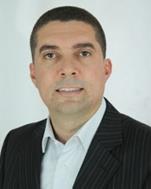
Artificial intelligence and its applications
Speaker 2: Prof. Hacéne BELBACHIR, University of Science and Technology Houari Boumediene, Algeria
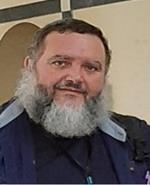
Graph theory and its applications
Bibliography: Hacene Belbachir has received the highest diploma in mathematics (D.E.S.), Option: Probability and statistics from the University of Science and Technology Houari Boumediene (USTHB). In 1991, he received the magister diploma in algebra and number theory from the USTHB, in 1994, the magister diploma in applied statistics and economy from the National Institute of Planification and Statistics (INPS, now ENSSEA), in 1996, and the doctorat d’Etat (PhD) degree, in mathematics on combinatorial number theory from the USTHB, in 2007. He was with the Department of Mathematics, USTHB since 1994 as an associate professor and from 2007 as full professor. Actually, he is the head of the Team Combinatory, Arithmetic and Theoretical Informatics (CATI Team), RECITS Laboratory, USTHB, associate member of LITIS Laboratory, Team Combinatory and Algorithms from Rouen University in France, and member of the Euro-Maghreb Laboratory of Mathematics and their Interactions (LEM2I).
Speaker 3:Prof. Eric MOULINES, Ecole Polytechnique, Paris, France
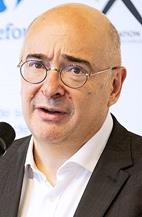
Divide-and-Conquer Posterior Sampling for Denoising Diffusion Priors
Interest in the use of denoising diffusion models (DDM) as priors for solving inverse Bayesian problems has recently increased significantly. However, sampling from the resulting posterior distribution poses a challenge. To address this problem, previous work has proposed approximations for the bias of the drift term of the diffusion. In this work, we take a different approach and utilize the specific structure of the DDM prior to define a set of intermediate and simpler posterior sampling problems, resulting in a lower approximation error compared to previous methods. We empirically demonstrate the reconstruction capability of our method for general linear inverse problems using synthetic examples and various image restoration tasks.
Bibliography:Eric Moulines earned a degree in engineering (1984) from the Ecole Polytechnique, and a PhD in electrical engineering (1990) from the Ecole Nationale Supérieure des Télécommunication. In 1990, he joined the Signal and Image Processing Department at Télécom ParisTech, where he was appointed full professor in 1996. In 2015, he joined the Centre for Applied Mathematics at Ecole Polytechnique, where he is currently Professor of Statistics. His areas of expertise include computational statistics (Monte Carlo simulations, stochastic optimization), probabilistic machine learning, statistical signal processing and time series analysis (sequential Monte Carlo, non-linear filtering). His current research topics include high-dimensional Monte Carlo sampling, stochastic optimization, and generative models (variational autoencoders, generative adversarial networks). He applies these different methods to uncertainty quantification, Bayesian inverse problems, and the control of complex systems. He has published more than 120 articles in leading journals in the areas of signal processing, computational statistics and applied probability and more than 300 proceedings papers in major signal processing and machine learning conferences. In 1997 and 2006, he received the Best paper Award from the IEEE Signal Processing Society (for publications in IEEE Trans. On Signal Processing). He has served on the editorial boards of IEEE Trans. On Signal Processing, Signal Processing, Stochastic Processes and Applications, Journal of Statistical Planning and Inference, Electronic Journal of Statistics. From 2013-2016, he was the Editor-in-Chief of Bernoulli. E. Moulines is a EURASIP and IMS Fellow. He was awarded the Silver Medal of the Centre National de Recherche Scientifique in 2010 and the Orange Prize of the French Academy of Sciences in 2011. In 2016, he was a Fellow of the IMS. In 2020, he received the technical achievement award from EURASIP. In 2017, he was elected to the Academy of Sciences.
Speaker 4:Prof. Karim ABED-MERAIM, University of Orleans, France
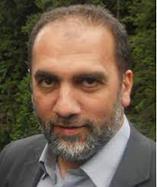
Tensor decomposition for neural network
Speaker 5:Prof. Mèrouane DEBBAH, Khalifa University, UAE
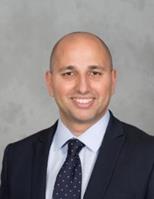
Large Language Models: Challenges and Opportunities
Bibliography: Mérouane Debbah is a researcher, educator and technology entrepreneur. Over his career, he has founded several public and industrial research centers, start-ups; and is now Professor at Khalifa University of Science and Technology in Abu Dhabi and founding Director of the Khalifa University 6G center. He is a frequent keynote speaker at international events in the field of telecommunication and AI. His research has been lying at the interface of fundamental mathematics, algorithms, statistics, information and communication sciences with a special focus on random matrix theory and learning algorithms. In the Communication field, he has been at the heart of the development of small cells (4G), Massive MIMO (5G) and Large Intelligent Surfaces (6G) technologies. In the AI field, he is known for his work on Large Language Models, distributed AI systems for networks and semantic communications. He received multiple prestigious distinctions, prizes and best paper awards (more than 35 best paper awards) for his contributions to both fields and according to research.com is ranked as the best scientist in France in the field of Electronics and Electrical Engineering. He is an IEEE Fellow, a WWRF Fellow, a Eurasip Fellow, an AAIA Fellow, an Institute Louis Bachelier Fellow and a Membre émérite SEE. His recent work led to the development of NOOR (upon it release, largest language model in Arabic) released in 2022 and Falcon LLM (upon its release, top ranked open source large language model) released in 2023. These two models have positioned the UAE as a global leader in the generative AI field.
Speaker 6: Prof. Adel BELOUCHRANI, Ecole Nationale Polytechnique, Algeria
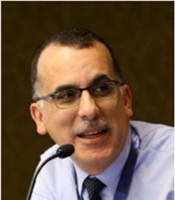
Source Separation: Model driven versus Data driven approaches
Speaker 7: Prof. Nguyen LINH TRUNG, VNU, Vietnam
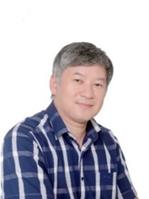
Signal and Information Processing Methods for Brain Studies
Recent advances in signal processing and machine learning have led to opportunities and challenges in brain studies. In this talk, we will present several research studies targeting three brain conditions: epilepsy, cognitive conflict, and Alzheimer’s disease. The first study, for epilepsy, develops a multi-stage system for single-channel EEG-based epileptic spike detection and a tensor decomposition method for multi-channel spike detection. The second study selects special computer-vision methods for EEG-based peak detection in cognitive conflict processing. The third study applies machine-learning methods for PET-based feature extraction and data-driven brain atlas construction in classification of Alzheimer’s disease. The fourth study applies machine-learning methods for MRI-based feature extraction in prediction of pathways between multivariate brain areas and multivariate disease/behavior outcomes.
Bibliography: Nguyen Linh Trung obtained his B.Eng. and Ph.D. degrees, both in Electrical Engineering, from Queensland University of Technology, Brisbane, Australia, in 1998 and 2005. He joined VNU University of Engineering and Technology, Vietnam National University, Hanoi (VNU), in 2006, where he is currently an associate professor of electronic engineering and founding director of the Advanced Institute of Engineering and Technology. His broad technical interests are theories, methods and applications of signal processing; in particular, detection and estimation, adaptive processing, subspace and tensor methods, blind separation, time-frequency analysis, graph processing, machine learning, and their applications in communications, networks and biomedicine. He has served as technical editor-in-chief of the Journal of Research and Development on Information and Communication Technology published by the Ministry of Information and Communication of Vietnam, founding chair of the IEEE Signal Processing Vietnam Chapter, founding chair of the Asia-Pacific Signal Processing and Information Vietnam Chapter general co-chair of the 2023 IEEE Statistical Signal Processing workshop.